Another marvelously instructive post from HASTAC scholar Tobias Hrynick, Department of History, Fordham University. Here he outlines some systematic tips and guidelines for creating a digitized version of a text.
The digital analysis of text constitutes the core of the digital humanities. It is here that Roberto Busa and his team began, and though subsequent scholars have expanded somewhat, exploring the possibilities of digital platforms for applying geographic analysis or presenting scholarship to wider audiences, the humanists’ interest in text has ensured the growth of a healthy trunk directly up from the root, along with the subsequent branches.
Necessary for all such projects at the outset, however, is the creation of a machine-readable text, on which digital analytical tools can be brought to bear. This process is generally more tedious than difficult, but it is nevertheless fundamental to digital scholarship, and a degree of nuance can be applied to it. What follows is intended as a basic introduction to some of the appropriate techniques, intended to highlight useful tools, including some (such as Antconc and Juxta) which also have powerful and user-friendly analytic functionality.
Acquiring Text
The appropriate way of acquiring a machine-readable text file (generally a .txt file, or some format which can be easily converted to .txt), and the difficulty involved in doing so, varies according to several factors. Often, digital versions of the text will already exist, so long as the text is old enough that the copyright has expired, or new enough that it was published digitally. Google Books, Project Gutenberg, and Archive.org all maintain substantial databases of free digital material. These texts, however, are all prone to errors – Google Books and Archive.org texts are generally created with a process of scanning and automated processing that is likely to produce just as many errors as performing this process yourself.
Such automated processing is called Optical Character Recognition (OCR). It requires a great deal of labor intensive scanning if you are working from a print book – though a purpose-built book scanner with a v-shaped cradle will speed the work considerably, and a pair of headphones will do a great deal to make the process more bearable.
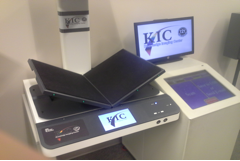
Once you have .pdf or other image files of all the relevant text pages, these files can be processed by one of a number of OCR software packages. Unfortunately, while freeware OCR software does exist, most of the best software is paid. Adobe Acrobat (not to be confused with the freely available Adobe Reader) is the most common, but another program, ABBYY Finereader deserves special mention for additional flexibility, particularly for more complicated page layouts, and a free trial version.
As a quick glance through the .html version of any Archive.org book will confirm, the outcome of an OCRing process is far from a clean copy. If a clean copy is required, you will need to expend considerable effort editing the text.
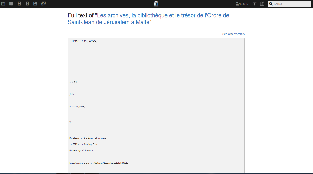
The other option is to simply re-type a given text in print or image format into a text editor – both Apple and Windows machines come with native text-editors, but if you are typing at length into such an editor, you might prefer a product like Atom or Notepad++. Neither of these platforms provides any crucial additional functionality, but both offer tabbed displays, which can be useful for editing multiple files in parallel; line numbers, which are helpful for quickly referencing sections of text; and a range of display options, which can make looking at the screen for long periods of time more pleasant. Alternately, you can choose to type out text in a word processor and then copy and paste it into a plain-text editor.
Assuming there is no satisfactory digital version of your text already available, the choice between scanning and OCRing, and manually retyping should be made keeping the following factors in mind:
- How long is your text?
This is important for two reasons. First, the longer a text is, the more the time advantage of OCR comes into play. Second, the longer a text is, the more individual errors within it become acceptable, which can sometimes make the time-consuming process of editing OCRed text by hand less critical. Digital textual analysis is best at making the king of broad arguments about a text in which a large sample size can insulate against the effects of particular errors.
The problem of this argument in favor of OCR is that it assumes the errors produced will be essentially random. OCR systems, however, when they make mistakes are likely to make that same mistake over and over again – particularly common are errors between the letters i, n, m, and r, and various combinations thereof – such errors are likely to cascade across the whole text file. A human typist might make more errors over the course of a text, especially a long text in a clear type-face, but the human is likely to make more random errors, which a large sample size can more easily render irrelevant.
That said, OCR should still generally be favored for longer texts. While automated errors can skew your results more severely than human ones, they are also more amenable to automated correction, as will be discussed in the next section.
- What is the quality of your print or image version?
Several features of a text which might cause a human reader to stumble only momentarily will cripple an OCR systems ability to render good text. Some such problems include:
- A heavily worn type-face.
- An unusual type-face (such as Fractur).
- Thin pages, with ink showing through from the opposite side.
If your text or image has any of these features, you can always try OCRing to check the extent of the problem, but it is wise to prepare yourself for disappointment and typing.
- How do you want to analyze the text?
Different kinds of study demand different textual qualities. Would you like to know how many times the definite article occurs relative to the indefinite article in the works of different writers? Probably, you don’t need a terribly high quality file to make such a study feasible. Do you want to create a topic model (a study of which words tend to occur together)? Accuracy is important, but a fair number of mistakes might be acceptable if you have a longer text. Do you intend to make a digital critical edition highlighting differences between successive printings of nineteenth century novels? You will require scrupulous accuracy. None of these possibilities totally preclude OCRing, especially for longer texts, but if you choose to OCR, expect a great deal of post-processing, and if the text is relatively short, you might be better served to simply retype it.
Cleaning Text
Once you have produced a digital text, either manually or automatically, there are several steps you can take to help reduce any errors you may have inadvertently introduced. Ultimately, there is no substitute for reading, slowly and out loud, by an experienced proof-reader. A few automated approaches, however, can help to limit the labor for this proof-reader or, if the required text quality is not high, eliminate the necessity altogether.
- Quick and Dirty: Quickly correcting the most prominent mistakes in an OCRed text file.
One good way of correcting some of the most blatant errors which may have been introduced, particularly the recurring errors which are common in the OCRing process, is with the use of concordancing software – software which generates a list of all the words which occur in a text. One such program is Antconc, which is available for free download, and contains a package of useful visualization tools as well.
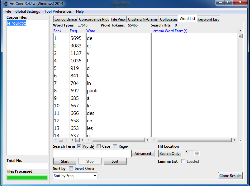
Once you have produced a text file, you can load it using AntConc, and click on the tab labeled Word List. This will produce a list of all the words occurring in the text, listed in order of frequency. Read through this list, noting down any non-words, or words whose presence in the text would be particularly surprising. Once you have noted down all the obvious and likely mistakes, you can correct them using the Find and Find and Replace tools on your preferred text editor.
This method of correction is far from fool-proof. Some subtle substitutions of one plausible word for another will likely remain. This is, however, a good way of quickly eliminating the most glaring errors from your text file.
A similar effect can be achieved using the spell-check and grammar check functions on a word processor, but there are several reasons the concordance method is generally preferable. First, reading through the list of words present in the text will tend to draw your attention to words which are real, but unlikely to be accurate readings in the context of the text, which would be over-looked by spelling and grammar-check functions. Second, a concordancer will present all the variants of a given word which occur in the text – through alternate spelling, use of synonyms, or varying grammatical forms (singular vs. plural, past vs. future) – which might be significant for your analysis.
- Slow and Clean: Cross-Collating multiple Text Files
A more extreme way of correcting digitized text is to produce multiple versions and to collate them together. Because of the frequency of similar errors being repeated across OCR versions, comparing two OCR versions is of limited use (although if you have access to more than one version of OCR software, it might be worth trying). It is of greater potential use if you compare two hand-typed versions, or a hand-typed version and an OCRed version, which are much less likely to contain identical errors.
Cross-comparison of two documents can be accomplished even using the merge document tools on Microsoft Word. A somewhat more sophisticated tool which can accomplish the same task is Juxta. This is an online platform (available also as a downloadable application), which is designed primarily to help produce editions from multiple varying manuscripts or editions, but which is just as effective as a way of highlighting errors which were introduced in the process of digitization.
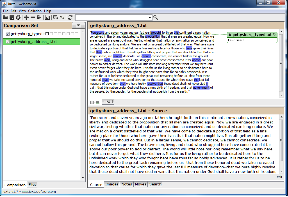
This process is a relatively thorough way of identifying errors in digitized text, which can even identify variations that might escape the attention of human proofreaders. The major weakness of the technique, however, is that it requires you to go through the effort of producing multiple different versions, ideally including one human-typed version. If you need a scrupulously corrected digital text, however, it is a powerful tool in your belt, and in the event that multiple digital versions of your text have already produced, it is an excellent way of using them in concert with one another – another strength of the Juxta platform is that you can collate together many different versions of the same text at once.
Conclusion
Once you have a digitized and cleaned version of the text in which you are interested, a world of possibilities opens up. At a minimum, you should be able to use computer search functions to quickly locate relevant sections within the text, while at maximum you might choose to perform complex statistical analysis, using a coding language like R or Python.
A good way to start exploring some of the possibilities of digital textual analysis is to go back and consider some of the tools associated with Antconc other than its ability to concordance a text. Antconc can be used to visualize occurrences of a word or phrase throughout a text, and to identify words which frequently occur together. Another useful tool for beginners interested in text analysis is Voyant, which creates topic models – visualizations of words which frequently occur together in the text, which can help to highlight key topics.